The automation of machine learning, particularly federated learning, is likely to become vitally important in the future of energy cybersecurity. This is especially true as cyber threats become ever more complex. New strategies such as federated learning and Adversarial Machine Learning (AML) are advancing the field by increasing the effectiveness of privacy-preserving threat detection and making it harder for cybercriminals to threaten ML systems. With federated learning, several energy organizations can train ML models without sensitive information being shared, thus enhancing confidentiality and making the entire industry more secure.
Enhancing Threat Detection with Machine Learning
Machine learning enhances threat detection by enabling systems to recognize attacks before they cause harm. With the new ML technologies, cyber threat security no longer needs to rely on set in stone rules and context, as the system is able to be more robust with identifying targets through supervised and deep learning techniques. These methods process and analyze large quantities of network traffic, filtering out the normal and observing the malicious. For example, sophisticated anomaly detection techniques could identify signs of malware attacks or suspicious activities, such as unauthorized access to grids.
ML models can improve the detection rate of sophisticated attacks such as Advanced Persistent Threats (APTs) and Distributed Denial-of-Service (DDoS) attacks by continuously learning from historical data. This ability to detect novel threats without requiring explicit programming makes ML indispensable in modern cybersecurity frameworks for energy systems.
Risk Mitigation and Automated Response Mechanisms
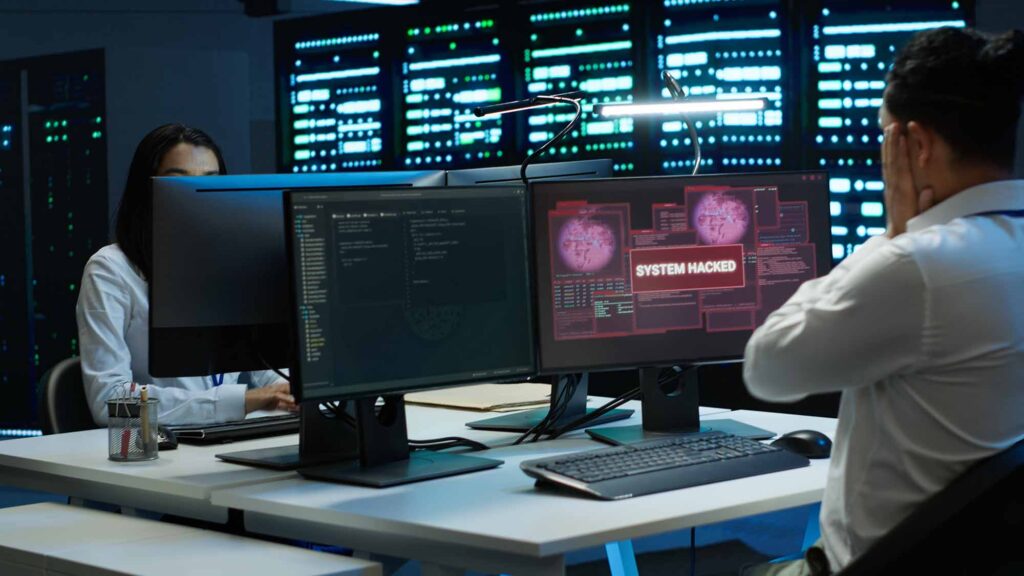
Beyond detection, machine learning significantly enhances risk mitigation strategies by automating threat response mechanisms. Energy infrastructures require real-time intervention to counter cyber threats, which ML-powered security systems facilitate through predictive analytics and automated decision-making. Key benefits include:
- Predictive Analytics: ML models analyze system behavior to forecast potential attack vectors and recommend preemptive security measures.
- Automated Decision-Making: Reinforcement learning algorithms dynamically adjust firewall rules and access controls to counter evolving threat landscapes.
- Adaptive Authentication: ML-driven authentication systems assess user behavior and grant or restrict access based on real-time risk evaluations.
- Reduced Downtime and Data Breaches: Automated responses help energy operators maintain system integrity, minimizing the impact of cyberattacks on critical infrastructure.
These proactive defenses ensure the resilience of energy assets against cyber threats by enabling rapid and intelligent threat mitigation.
The Future of Machine Learning in Energy Cybersecurity
The automation of machine learning, particularly federated learning, is likely to become vitally important in the future of energy cybersecurity. This is especially true as cyber threats become ever more complex. Emerging AI-driven approaches such as federated learning and adversarial machine learning are advancing the field by increasing the effectiveness of privacy-preserving threat detection and making it harder for cybercriminals to threaten ML systems. With federated learning, several energy organizations can train ML models without sensitive information being shared, thus enhancing confidentiality and making the entire industry more secure.
In a similar way, AML strengthens security AI by weakening Machine learning models and subjecting them to simulated cyberattacks, which helps devise stronger detection and mitigation techniques. In the context of security of distributed energy networks, the fusion of machine learning algorithms and blockchain technology has a significant effect. It allows reliable, tamper-proof recording of cyber incidents together with the corresponding response measures. Hence, it is crucial to incorporate developments in machine learning, artificial intelligence, and cybersecurity to ensure the safety, reliability, and modern power systems in the energy sector.
Conclusion
Machine learning has emerged as an essential component in shaping the energy operation landscape by defining how threats are identified, detected and mitigated. ML-based solutions help in automating risk mitigation and improving threat detection, in turn influencing the future of cybersecurity. With cyber threats evolving in complexity, integrating devices that employ machine learning will be critical to improving existing systems, anticipating issues, and enabling advanced measures to maintain security. For energy providers that seek robust ways to protect their networks from a converging array of threats, ML-based solutions provide the missing piece of the puzzle.
Disclaimer: Any opinions expressed in this blog do not necessarily reflect the opinions of Certrec. This content is meant for informational purposes only.